Stat Analysis For Injury Risk offers a proactive approach to minimizing harm in sports and physical activities by identifying patterns and predictors. This article explores how data analytics is revolutionizing injury prevention strategies, covering key statistical methods, real-world applications, and the ethical considerations involved.
⚠️ Still Using Pen & Paper (or a Chalkboard)?! ⚠️
Step into the future! The Dart Counter App handles all the scoring, suggests checkouts, and tracks your stats automatically. It's easier than you think!
Try the Smart Dart Counter App FREE!Ready for an upgrade? Click above!
Understanding the Importance of Stat Analysis For Injury Risk
The realm of sports and physical activity is one of peak performance and dedication, but it also carries inherent risks. Injuries can sideline athletes, impact careers, and lead to long-term health issues. Traditionally, injury prevention relied heavily on anecdotal evidence and general guidelines. However, the rise of data analytics has opened new avenues for a more scientific and personalized approach. Stat Analysis For Injury Risk offers a powerful toolset for understanding the complex factors that contribute to injuries, allowing for targeted interventions and improved safety protocols.
By leveraging statistical methods, we can move beyond reactive treatment and embrace proactive prevention. This shift not only benefits athletes but also has implications for occupational health, rehabilitation programs, and even everyday activities where injury is a concern. The ability to predict and mitigate risk is transforming how we approach physical well-being.
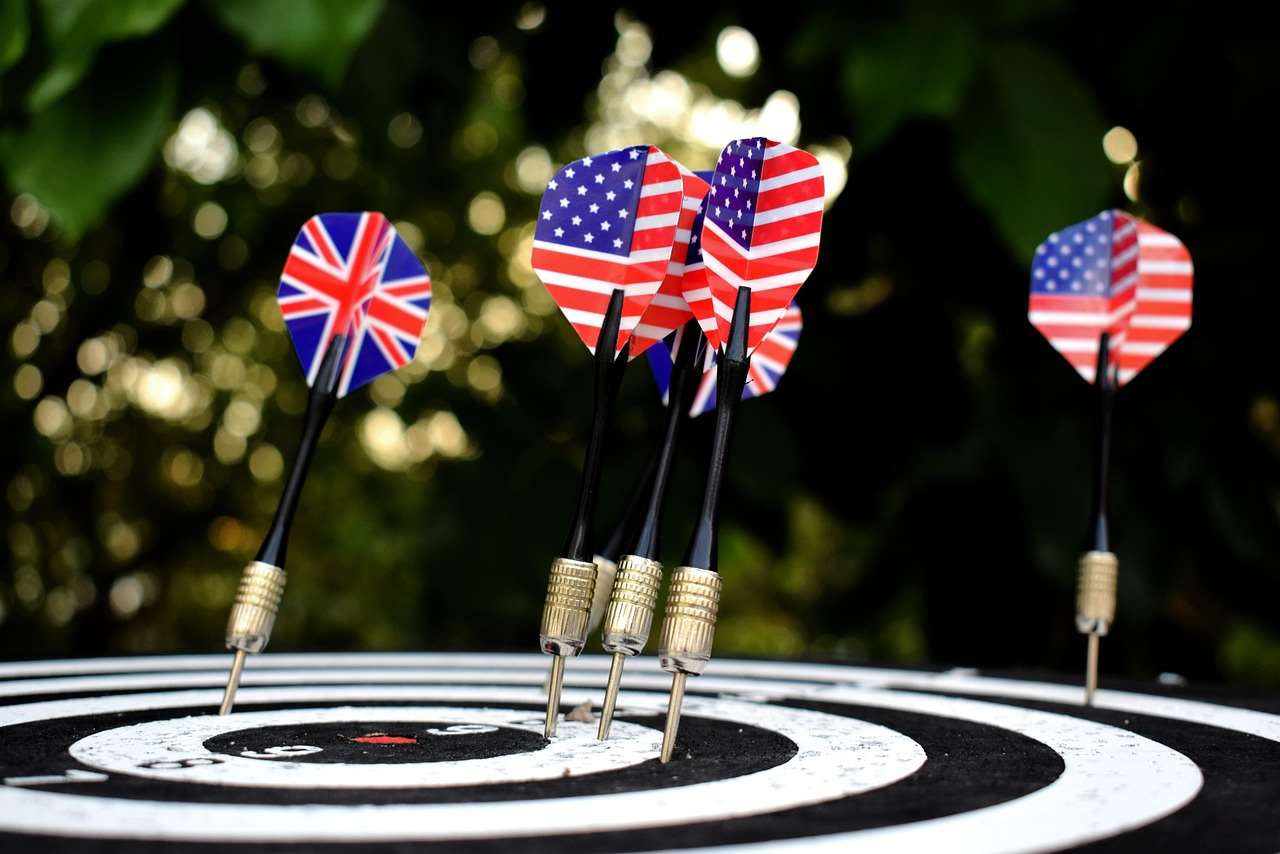
Key Statistical Methods Used in Injury Risk Assessment
Several statistical methods are commonly employed in stat analysis for injury risk. Each technique offers unique insights, and often a combination of methods provides the most comprehensive understanding.
- Descriptive Statistics: This foundational approach involves summarizing and presenting data in a meaningful way. Measures like mean, median, mode, and standard deviation are used to characterize injury rates, severity, and patterns across different populations or activities. For example, calculating the average number of injuries per season in a particular sport provides a baseline for comparison and helps identify trends.
- Regression Analysis: This technique examines the relationship between dependent variables (e.g., injury occurrence) and independent variables (e.g., training load, age, gender, biomechanics). Regression models can identify which factors are most strongly associated with injury risk, allowing for targeted interventions. Both linear and logistic regression are frequently used, depending on the nature of the data.
- Survival Analysis: Also known as time-to-event analysis, this method focuses on the time it takes for an event (in this case, an injury) to occur. It’s particularly useful for comparing injury rates between different groups or interventions. Survival curves can illustrate how the probability of injury changes over time, providing valuable insights for injury prevention strategies.
- Machine Learning: These algorithms can identify complex patterns and relationships in data that may not be apparent through traditional statistical methods. Techniques like decision trees, support vector machines, and neural networks are increasingly being used to predict injury risk based on a wide range of factors. Machine learning models can also be used to personalize injury prevention strategies based on individual risk profiles. For instance, a machine learning algorithm might identify a unique combination of factors that significantly increases the risk of ACL tears in female athletes.
- Bayesian Statistics: This approach incorporates prior knowledge or beliefs into the analysis, allowing for more informed decision-making. Bayesian methods are particularly useful when dealing with limited data or when incorporating expert opinions into the analysis. For example, prior research on the effectiveness of a particular injury prevention program can be incorporated into a Bayesian model to estimate the program’s effectiveness in a new population.
Understanding these methods is crucial for interpreting and applying stat analysis for injury risk effectively. Choosing the appropriate method depends on the research question, the type of data available, and the desired level of precision.
Choosing the Right Statistical Method
The selection of a statistical method is paramount to accurate injury risk assessment. Each technique caters to specific data types and research questions. For instance, if the aim is to establish a relationship between training intensity and injury rate, regression analysis might be the most suitable choice. Conversely, if the goal is to predict the time until an athlete sustains an injury, survival analysis would be more appropriate. Consulting with a statistician or data analyst can significantly enhance the rigor and relevance of your analysis.
Furthermore, the quality and completeness of the data are critical. Garbage in, garbage out, as they say. Accurate and consistent data collection practices are essential for reliable results. Consider investing in robust data management systems and training personnel in proper data entry techniques. Regular audits of data quality can also help identify and correct errors.
Real-World Applications of Injury Risk Analytics
The power of stat analysis for injury risk lies in its practical applications. Here are some examples of how this approach is being used in various settings:
- Sports Teams: Professional and amateur sports teams are increasingly using data analytics to monitor player workload, identify fatigue, and predict injury risk. Wearable sensors, such as GPS trackers and accelerometers, provide real-time data on player movement and physiological responses. This data is then analyzed to identify players who are at high risk of injury and to adjust training schedules accordingly. Predictive analytics can help optimize training regimens and minimize downtime due to injuries.
- Occupational Health: In industries with high physical demands, such as construction and manufacturing, stat analysis for injury risk is used to identify ergonomic hazards and implement preventive measures. Data on worker movements, postures, and environmental conditions is collected and analyzed to identify risk factors for musculoskeletal disorders and other work-related injuries. This information can then be used to redesign workstations, implement training programs, and provide workers with appropriate safety equipment. Building Local Darts League Club Guide can be a valuable asset.
- Rehabilitation Programs: Data analytics is also playing a growing role in rehabilitation programs. By tracking patient progress and identifying factors that contribute to successful recovery, clinicians can personalize treatment plans and improve outcomes. Data on patient demographics, medical history, and functional abilities is analyzed to predict the likelihood of successful rehabilitation and to identify potential barriers to recovery. This information can then be used to tailor treatment strategies to individual patient needs.
- Military Training: The military utilizes injury risk analysis to optimize training programs and reduce injuries among recruits. Analyzing data related to physical fitness tests, training routines, and environmental conditions helps identify patterns that contribute to injuries. This allows for adjustments to training protocols, ensuring that recruits are adequately prepared for the demands of military service while minimizing the risk of injury.
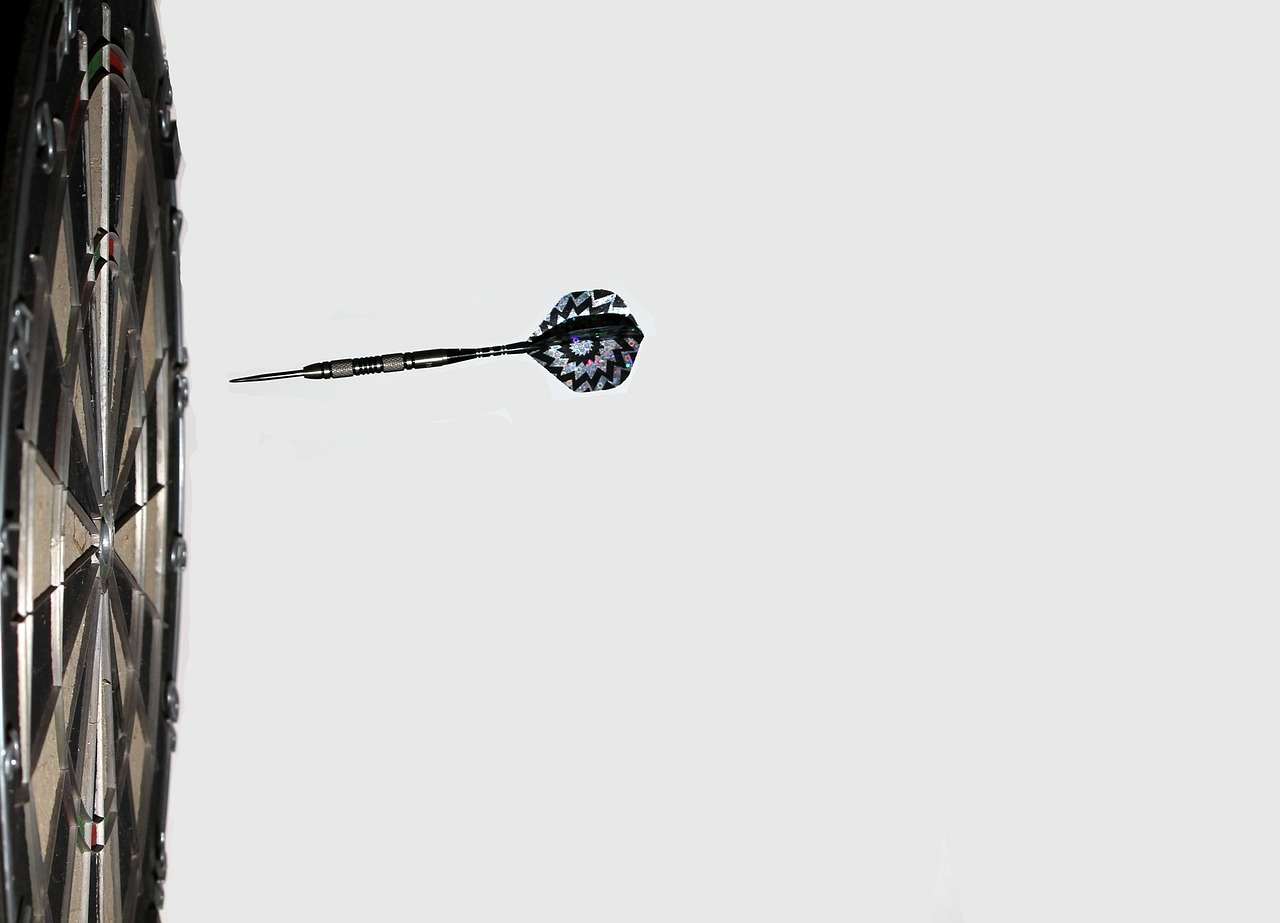
Predictive Modeling: The Future of Injury Prevention
Predictive modeling is becoming increasingly sophisticated, leveraging advanced algorithms and larger datasets to anticipate potential injuries before they occur. By analyzing historical injury data, player biometrics, training loads, and even environmental factors, these models can identify individuals at elevated risk and recommend targeted interventions.
For example, a predictive model might flag an athlete who is exhibiting early signs of fatigue, based on changes in their movement patterns and physiological responses. The coaching staff can then adjust the athlete’s training schedule to prevent overtraining and reduce the risk of injury. Similarly, in an occupational setting, a predictive model might identify workers who are at high risk of developing carpal tunnel syndrome, based on their job tasks and ergonomic risk factors. The employer can then implement ergonomic interventions to reduce the risk of injury.
The Role of Wearable Technology
The proliferation of wearable technology has significantly enhanced the capabilities of stat analysis for injury risk. Devices like smartwatches and fitness trackers provide a continuous stream of data on an individual’s activity levels, sleep patterns, and physiological metrics. This real-time data can be integrated into predictive models, providing a more comprehensive and personalized assessment of injury risk. Wearable sensors can detect subtle changes in movement patterns and physiological responses that might indicate early signs of fatigue or overtraining, allowing for timely interventions to prevent injuries.
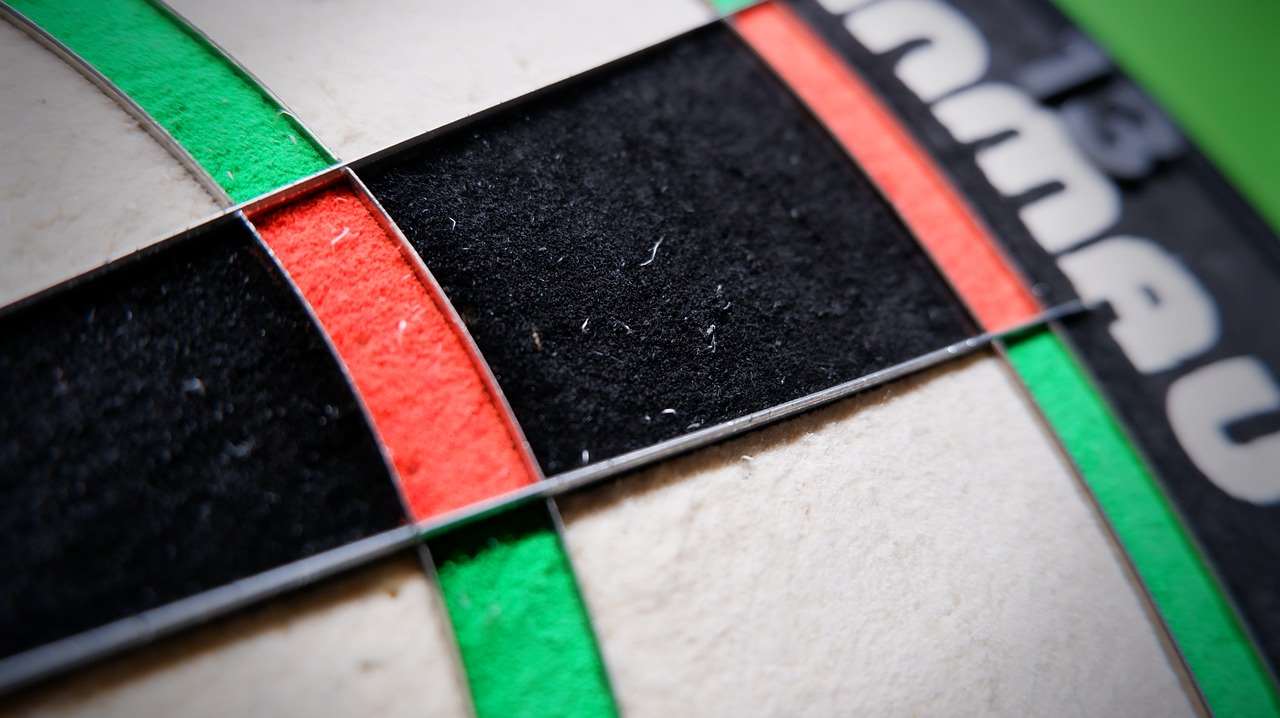
Challenges and Ethical Considerations in Stat Analysis For Injury Risk
While stat analysis for injury risk offers tremendous potential, it also presents several challenges and ethical considerations that must be addressed. One key challenge is data privacy. Collecting and analyzing sensitive personal data raises concerns about the security and confidentiality of that information. It’s crucial to implement robust data protection measures and to ensure that individuals are informed about how their data is being used.
Another challenge is data bias. If the data used to train predictive models is biased, the models may perpetuate existing inequalities. For example, if a model is trained primarily on data from male athletes, it may not accurately predict injury risk in female athletes. It’s essential to carefully consider the potential for bias in the data and to take steps to mitigate its effects. Another important aspect is Darts Culture And Community Guide.
Ethical considerations also arise regarding the use of predictive models to make decisions about individuals. For example, should an athlete be excluded from competition based on a predictive model that suggests they are at high risk of injury? It’s important to balance the potential benefits of injury prevention with the individual’s right to participate in activities they enjoy. Transparency and fairness are crucial in these situations.
- Data Privacy: Implement robust data security measures and obtain informed consent.
- Data Bias: Ensure data is representative and address potential biases in the models.
- Transparency: Be transparent about how the models work and how they are used.
- Fairness: Avoid using models that discriminate against certain groups.
Practical Tips for Implementing Stat Analysis For Injury Risk
Implementing effective stat analysis for injury risk requires a strategic approach and a commitment to data-driven decision-making. Here are some practical tips to help you get started:
- Define Clear Objectives: Start by clearly defining your goals. What specific injuries are you trying to prevent? What metrics will you use to measure success? Having clear objectives will help you focus your efforts and ensure that you’re collecting the right data.
- Collect High-Quality Data: The quality of your data is crucial. Invest in robust data collection systems and train your staff on proper data entry techniques. Ensure that your data is accurate, complete, and consistent.
- Choose the Right Statistical Methods: Select statistical methods that are appropriate for your research questions and the type of data you have. Consult with a statistician or data analyst if you’re unsure which methods to use.
- Interpret Results Carefully: Don’t jump to conclusions based on statistical analysis alone. Consider the context of your data and the limitations of the methods you used. Seek expert advice when interpreting complex results.
- Implement Interventions: Use the insights from your analysis to implement targeted interventions. Monitor the effectiveness of these interventions and adjust your strategies as needed.
- Foster a Data-Driven Culture: Encourage a culture of data-driven decision-making throughout your organization. Make data accessible to those who need it and provide training on how to interpret and use data effectively.
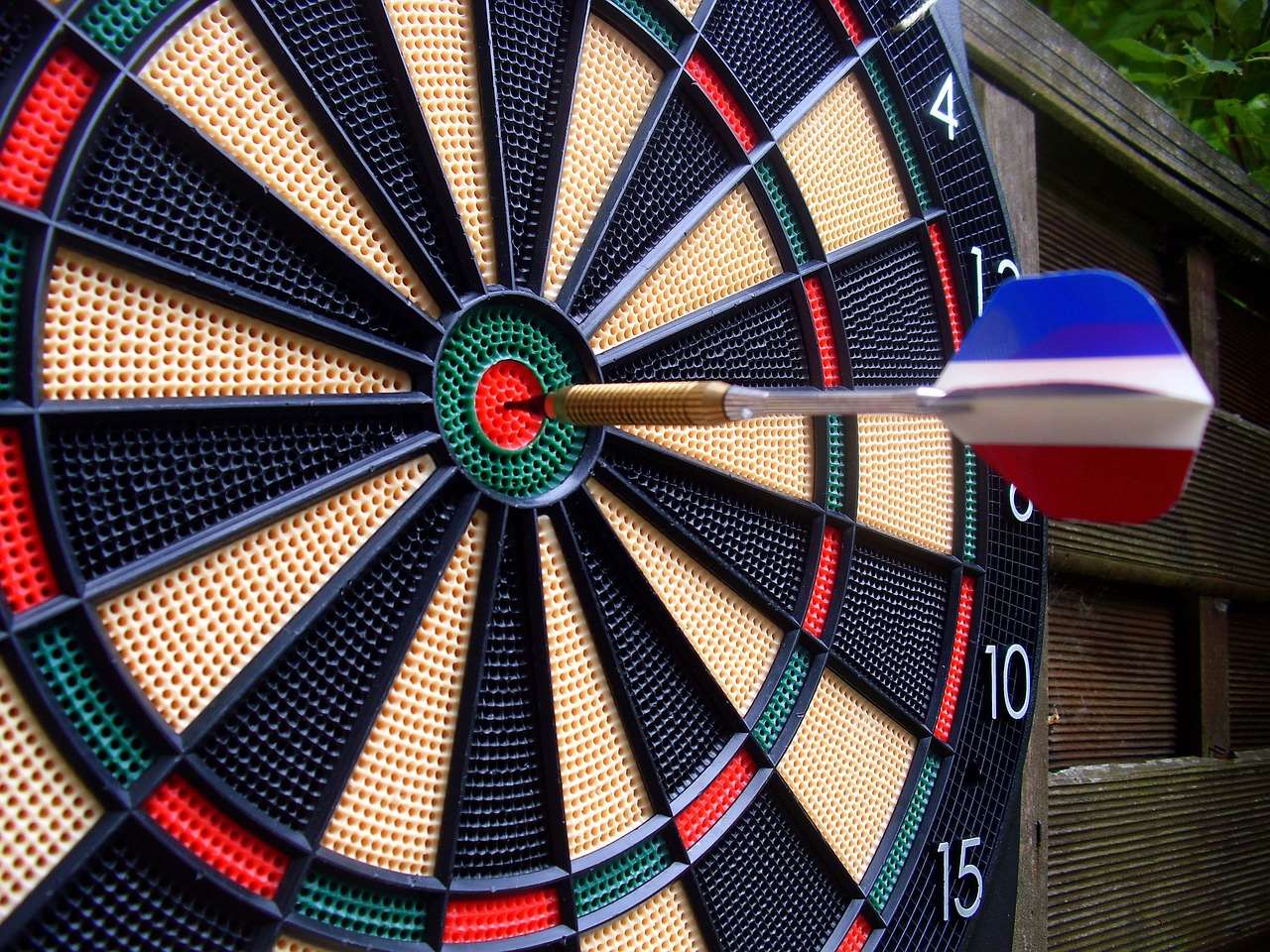
The Future of Injury Prevention: Integrated and Personalized Approaches
The future of injury prevention lies in integrated and personalized approaches that combine stat analysis for injury risk with other technologies and interventions. This includes:
- Integrating data from multiple sources: Combining data from wearable sensors, electronic health records, and environmental monitoring systems can provide a more comprehensive picture of an individual’s risk factors.
- Developing personalized injury prevention programs: Tailoring interventions to individual needs and risk profiles can improve effectiveness.
- Using artificial intelligence to automate data analysis and decision-making: AI can help identify patterns and relationships in data that might be missed by human analysts.
- Promoting collaboration between researchers, clinicians, and athletes: Working together can ensure that research findings are translated into practical applications.
By embracing these integrated and personalized approaches, we can create a future where injuries are less common and individuals can participate in physical activities safely and confidently.
Resources for Learning More About Stat Analysis For Injury Risk
There are numerous resources available for those interested in learning more about stat analysis for injury risk. These include:
- Academic Journals: Publications like the *American Journal of Sports Medicine* and the *Journal of Occupational and Environmental Medicine* regularly feature research on injury prevention and data analytics.
- Online Courses: Platforms like Coursera and edX offer courses on statistics, data analysis, and machine learning that can be applied to injury risk assessment.
- Professional Organizations: Organizations like the National Athletic Trainers’ Association (NATA) and the American Society of Safety Professionals (ASSP) offer resources and training on injury prevention and workplace safety.
- Books: Several books provide comprehensive overviews of statistical methods and their applications in injury prevention.
Continuing education and staying abreast of the latest research are crucial for professionals working in this field. Consider attending conferences, workshops, and webinars to expand your knowledge and network with other experts. Remember to Organizing Local Darts League to help people come together.
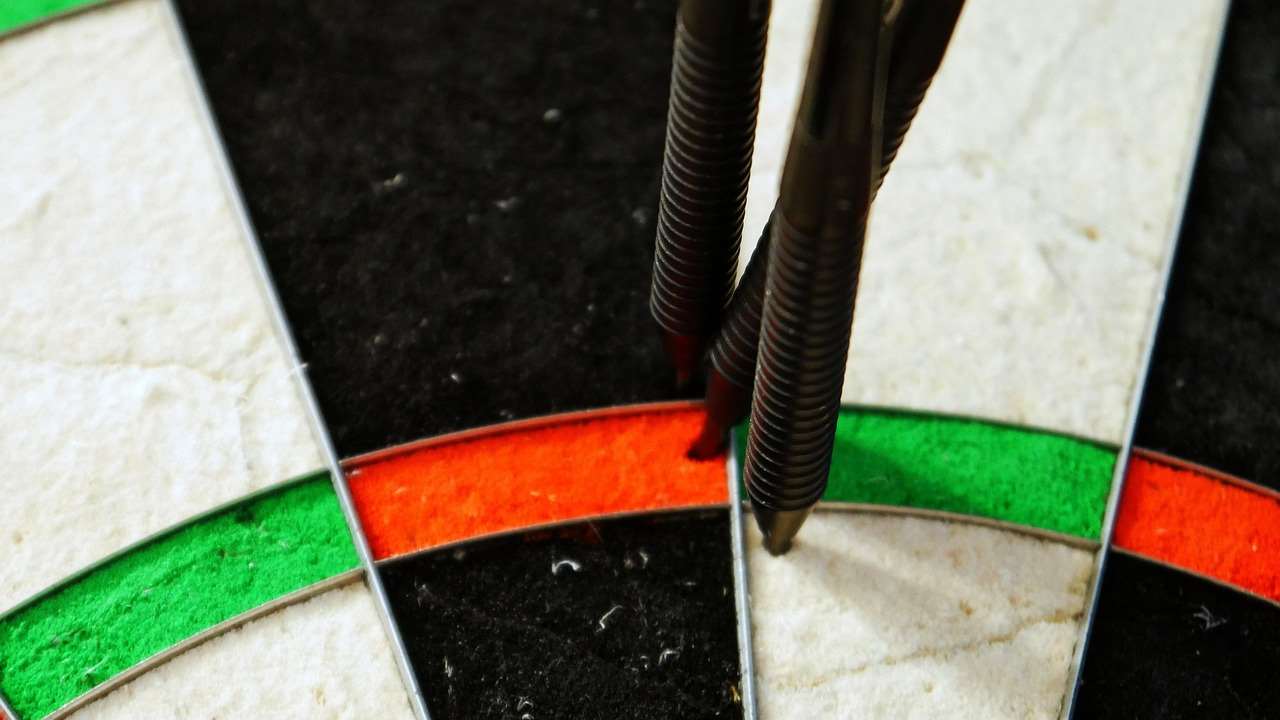
Conclusion: Embracing Data-Driven Injury Prevention
Stat Analysis For Injury Risk is transforming the way we approach injury prevention in sports, occupational health, and rehabilitation. By leveraging statistical methods, we can identify risk factors, predict injuries, and implement targeted interventions to improve safety and well-being. While challenges and ethical considerations exist, the potential benefits of data-driven injury prevention are undeniable. Embrace the power of data, stay informed about the latest research, and work collaboratively to create a future where injuries are less common and individuals can participate in physical activities safely and confidently. Learn How To Start A Darts League. By taking a proactive approach to **injury risk assessment**, we can create a healthier and safer environment for everyone.
Ready to implement stat analysis for injury risk in your organization? Contact us today for a consultation and discover how we can help you reduce injuries, improve performance, and enhance well-being.
Hi, I’m Dieter, and I created Dartcounter (Dartcounterapp.com). My motivation wasn’t being a darts expert – quite the opposite! When I first started playing, I loved the game but found keeping accurate scores and tracking stats difficult and distracting.
I figured I couldn’t be the only one struggling with this. So, I decided to build a solution: an easy-to-use application that everyone, no matter their experience level, could use to manage scoring effortlessly.
My goal for Dartcounter was simple: let the app handle the numbers – the scoring, the averages, the stats, even checkout suggestions – so players could focus purely on their throw and enjoying the game. It began as a way to solve my own beginner’s problem, and I’m thrilled it has grown into a helpful tool for the wider darts community.