Understanding how to **Stat Analysis Mistakes Avoid** is crucial for drawing accurate conclusions and making informed decisions; failing to do so can lead to flawed research and ineffective strategies. This article will explore common pitfalls in statistical analysis and provide practical guidance to help you avoid them, covering everything from data collection to interpretation and reporting.
⚠️ Still Using Pen & Paper (Of een schoolbord)?! ⚠️
Stap in de toekomst! De Dart Teller -app behandelt alle scoren, stelt kassa voor, en volgt uw statistieken automatisch. It's easier than you think!
Probeer de Smart Dart Teller -app gratis!Klaar voor een upgrade? Klik hierboven!
Common Data Collection & Preparation Errors
The foundation of any sound statistical analysis is the quality of the data itself. Errors in data collection and preparation can propagate throughout the entire process, leading to misleading or even entirely incorrect results. Ignoring the importance of meticulous data handling is one of the most frequent **stat analysis mistakes avoid**. Before diving into analysis, consider these points.
Sampling Bias: Skewing Your Results
Sampling bias occurs when the sample selected for analysis is not representative of the population you’re trying to study. This can happen in many ways. Bijvoorbeeld, if you’re surveying customer satisfaction by only interviewing customers who voluntarily call in to complain, you’re likely to get a skewed picture of overall satisfaction. Similarly, conducting a study during a specific time of year could unintentionally introduce seasonal bias, especially if the study involves human behavior or consumer preferences. To combat sampling bias:
- Ensure random sampling techniques are implemented properly.
- Clearly define the target population.
- Consider stratification to ensure representation from different subgroups within the population.
Data Cleaning Oversights: Missing Values and Outliers
Once the data is collected, it needs to be cleaned. Overlooking issues such as missing values En outliers is a common **stat analysis mistakes avoid**. Missing values can significantly impact statistical tests, while outliers can disproportionately influence results, especially in smaller datasets. Strategies for addressing these issues include:
- Imputation: Replacing missing values with estimated values (Bijv., mean, median, or mode). The choice of imputation method should be justified based on the nature of the data and the amount of missingness.
- Outlier Detection: Using statistical techniques like the interquartile range (IQR) or z-scores to identify and investigate potential outliers.
- Robust Statistical Methods: Employing statistical methods that are less sensitive to outliers.
Remember to document all data cleaning steps thoroughly so your analysis is reproducible.
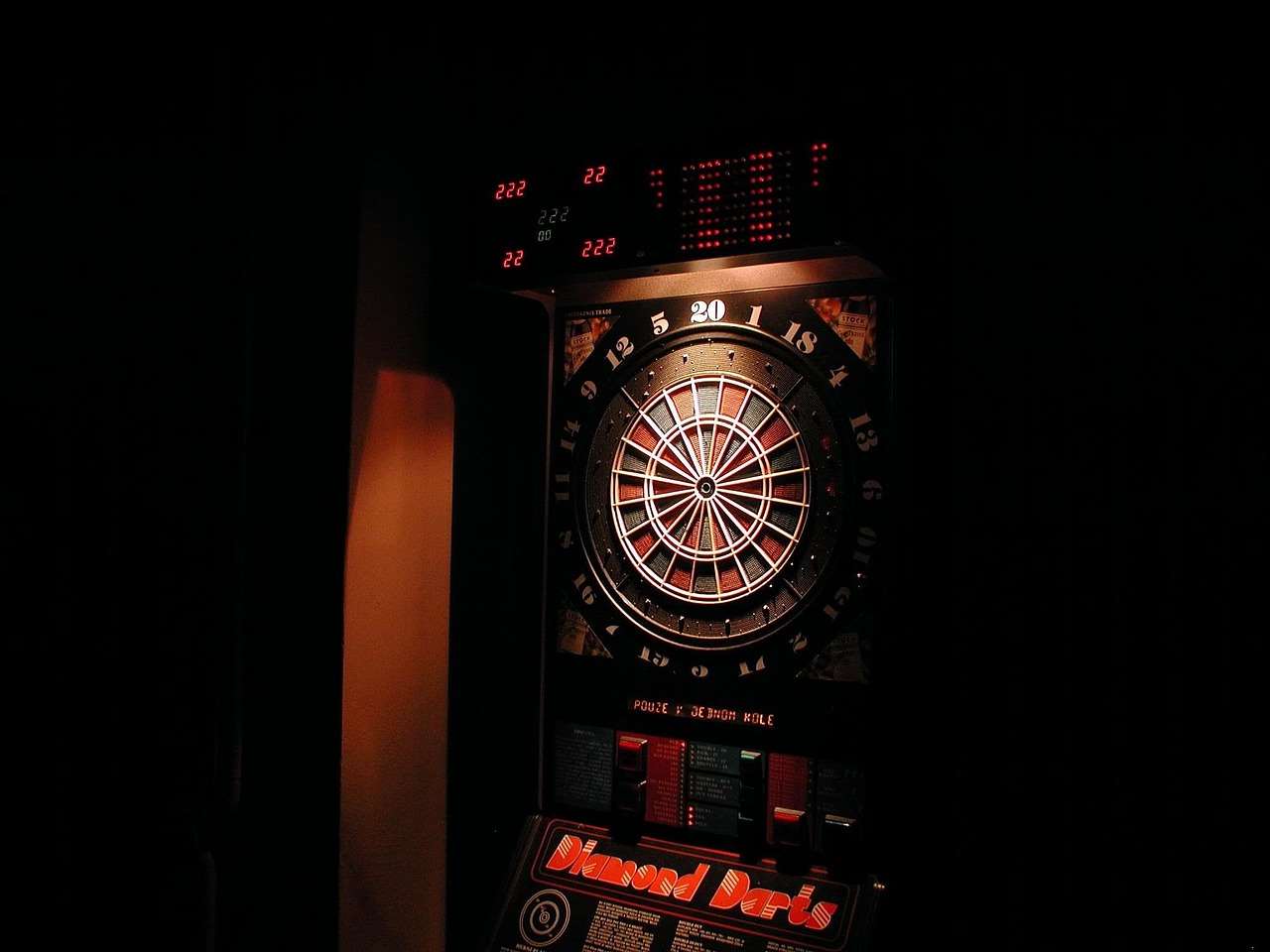
Choosing the Wrong Statistical Test
One of the most critical aspects of statistical analysis is selecting the appropriate test for your data and research question. Using the wrong test can lead to incorrect conclusions, no matter how meticulously the data was collected. Understanding the assumptions and limitations of different statistical tests is essential to **stat analysis mistakes avoid**.
Ignoring Assumptions of Statistical Tests
Every statistical test has certain assumptions about the data it’s designed to analyze. These assumptions might relate to the distribution of the data (Bijv., normality), the independence of observations, or the equality of variances. Failing to check these assumptions can invalidate the results of the test. Bijvoorbeeld:
- T-tests assume that the data is normally distributed and that the variances of the two groups being compared are equal.
- ANOVA (Analysis of Variance) also assumes normality and homogeneity of variances.
- Regression analysis assumes linearity, independence of errors, homoscedasticity (constant variance of errors), and normality of errors.
Before running a statistical test, always verify that its assumptions are met. If not, consider transforming the data or using a non-parametric alternative. Begrijpen hoe Darts gok en fantasiecompetities gids work helps in recognizing statistical patterns and applying the correct tests.
Confusing Correlation and Causation
This is a classic pitfall in statistical analysis. Just because two variables are correlated doesn’t mean that one causes the other. There might be a third, confounding variable that influences both, or the relationship might be purely coincidental. Bijvoorbeeld, ice cream sales and crime rates might be correlated, but this doesn’t mean that eating ice cream causes crime. In plaats van, both might be influenced by warmer weather. To avoid this:
- Consider potential confounding variables.
- Use controlled experiments to establish causation.
- Be cautious when interpreting correlational studies.
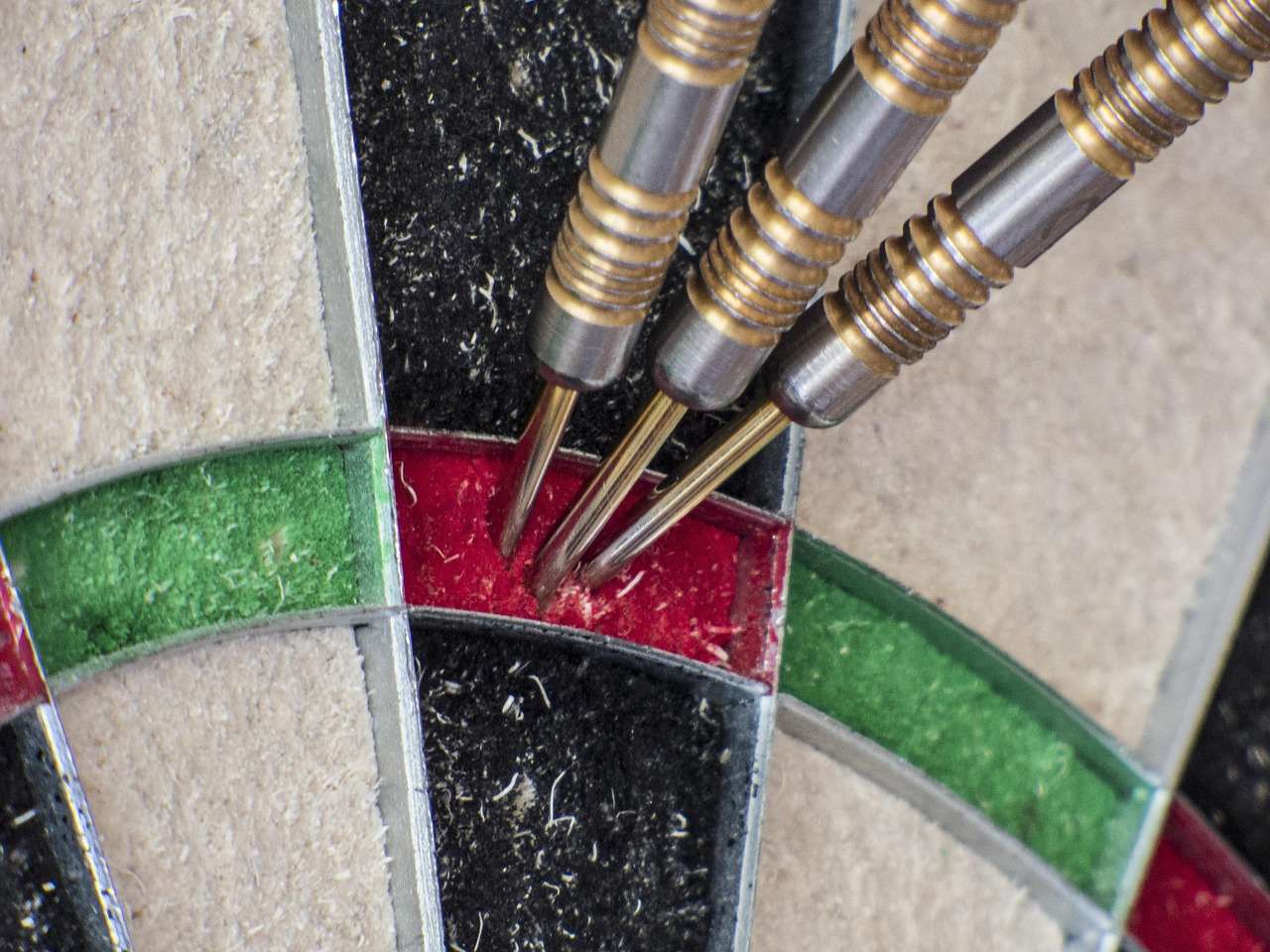
Overfitting and Data Dredging
Two related but distinct problems in statistical analysis are overfitting and data dredging. Both can lead to models that perform well on the data used to build them but generalize poorly to new data. Avoiding these issues is a key part of the work to **Stat Analysis Mistakes Avoid**.
Overfitting: Creating a Model That’s Too Complex
Overfitting occurs when a statistical model is too complex and fits the noise in the data rather than the underlying signal. This can happen when you include too many predictor variables in a regression model or when you use a highly flexible machine learning algorithm. An overfitted model will perform well on the training data but poorly on new, unseen data. To prevent overfitting:
- Use simpler models with fewer parameters.
- Employ regularization techniques (Bijv., L1 or L2 regularization).
- Use cross-validation to assess model performance on unseen data.
Data Dredging (P-Hacking): Searching for Significance Where None Exists
Data dredging, also known as p-hacking, involves repeatedly testing different hypotheses until you find one that is statistically significant. This increases the likelihood of finding spurious correlations and drawing incorrect conclusions. Common p-hacking practices include:
- Trying multiple statistical tests until one yields a significant p-value.
- Adding or removing variables from a model until a desired result is achieved.
- Analyzing subgroups of the data until a significant effect is found.
To avoid data dredging:
- Clearly define your research question and hypotheses before analyzing the data.
- Use a pre-registration protocol to specify your analysis plan in advance.
- Correct for multiple testing using techniques like the Bonferroni correction or the False Discovery Rate (FDR) control.
Pre-registration helps ensure that your analysis is driven by your research question rather than by the data itself.
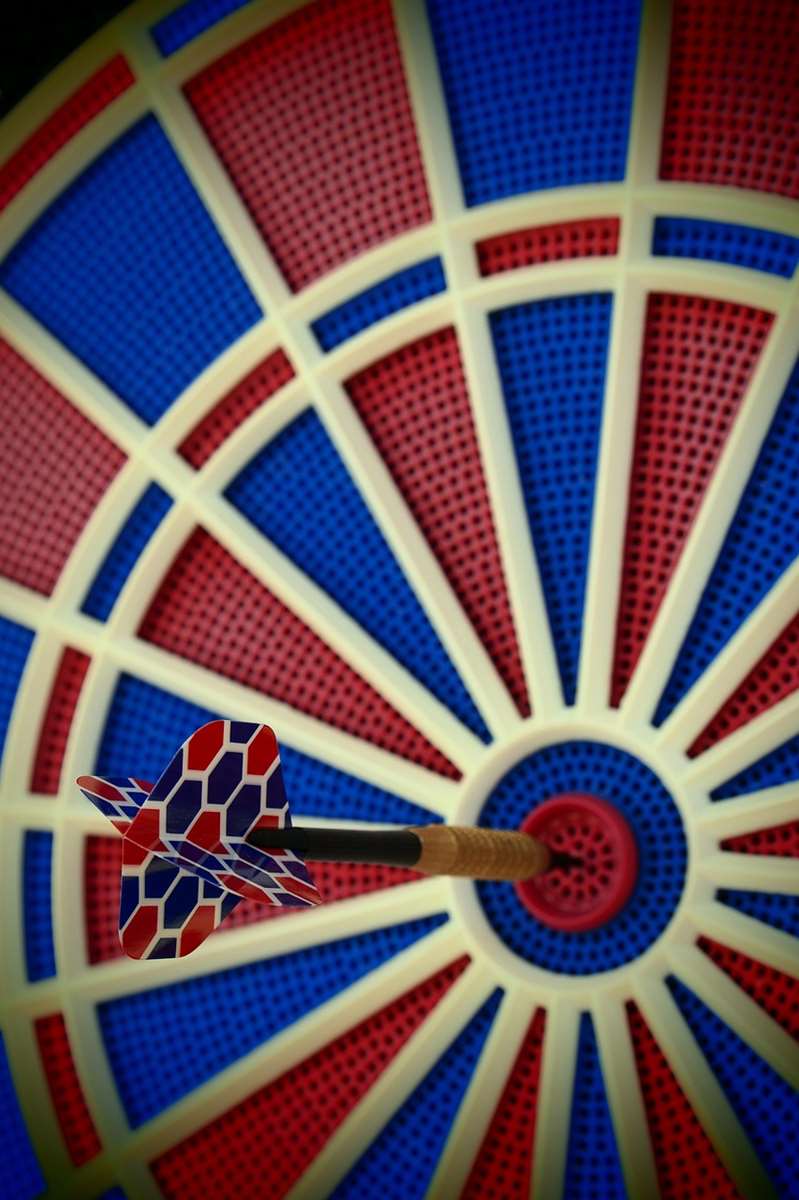
Interpretation and Reporting Errors
Even if the statistical analysis is performed correctly, errors can still occur in the interpretation and reporting of the results. Misinterpreting p-values, exaggerating the magnitude of effects, and failing to report limitations are all common **stat analysis mistakes avoid**.
Misinterpreting P-Values and Statistical Significance
A p-value is the probability of observing the data (or more extreme data) if the null hypothesis is true. A small p-value (typically less than 0.05) is often interpreted as evidence against the null hypothesis. Echter, it’s crucial to understand what a p-value does not mean. A p-value does not indicate the probability that the null hypothesis is false, nor does it measure the size or importance of an effect. Emphasizing clear and correct reporting helps clarify Betting Company Logos Darts Boards and the impact they may have.
Statistical significance does not necessarily imply practical significance. A statistically significant result may be too small to be meaningful in the real world. It is important to consider the effect size and the context of the research when interpreting p-values.
Exaggerating the Magnitude of Effects
When reporting statistical results, it’s important to avoid exaggerating the magnitude of effects. Bijvoorbeeld, if you find a statistically significant difference between two groups, don’t overstate the practical importance of that difference. Provide context and consider the effect size. Use confidence intervals to provide a range of plausible values for the effect.
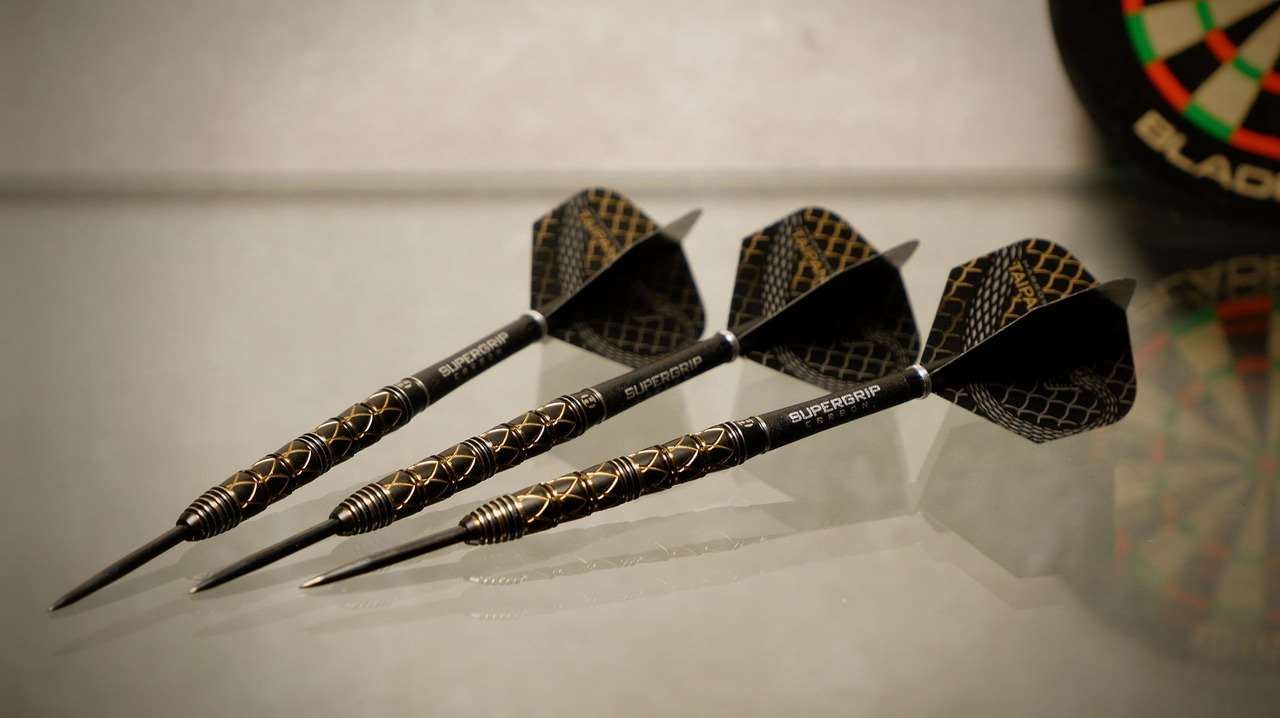
Failing to Report Limitations
No study is perfect, and every study has limitations. Failing to acknowledge these limitations can undermine the credibility of your research. Be transparent about potential sources of bias, limitations in the data, and assumptions that were made during the analysis. A thorough discussion of limitations shows that you’ve critically evaluated your own work. Considering the broader Betting Sponsorship Media Coverage helps frame data accuracy.
Moreover, be mindful of how statistical power impacts your study. Low statistical power (the probability of correctly rejecting a false null hypothesis) can lead to false negatives. If your study has low power, it may fail to detect a real effect. It’s essential to consider statistical power during the design phase of your study and to report it along with your results. Reporting Bookmaker Sponsorship Professional Darts properly also requires transparency.
Using Statistical Software Incorrectly
Statistical software packages are powerful tools, but they can also be a source of errors if used incorrectly. Common pitfalls include using the wrong commands, misinterpreting the output, and failing to validate the results. These are key **stat analysis mistakes avoid**.
Incorrect Command Usage and Syntax Errors
Statistical software packages have specific syntax rules and commands that must be followed precisely. Even a minor typo can lead to errors or unexpected results. Always double-check your code and syntax before running your analysis. Make use of the documentation and tutorials provided by the software vendor. Begrip Weddenschap sponsoring impact op darts can guide better decision-making in data applications.
Misinterpreting Software Output
Statistical software produces a wealth of output, including tables, graphs, and diagnostic statistics. It’s crucial to understand what each element of the output means and how to interpret it correctly. Don’t blindly accept the software’s results without critically evaluating them.
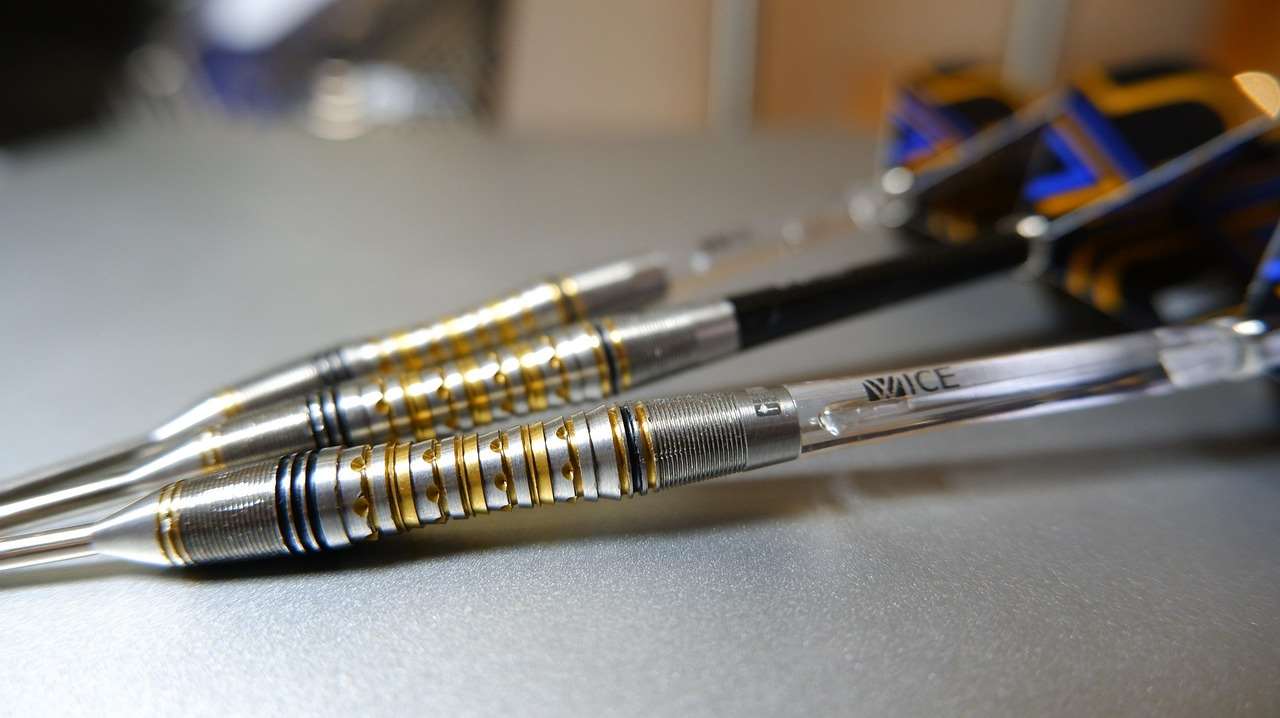
Failing to Validate Results
It’s good practice to validate your statistical results using multiple methods. Bijvoorbeeld, you can try running the same analysis using different software packages or different statistical techniques. You can also compare your results to those of other studies in the literature. Validating your results increases your confidence in their accuracy. Herinneren, consistently working to **stat analysis mistakes avoid** is a long-term strategy.
Conclusie
Avoiding statistical analysis errors requires a comprehensive approach that encompasses careful data collection, appropriate test selection, rigorous analysis, and accurate interpretation. By being aware of the common pitfalls discussed in this article and implementing strategies to mitigate them, you can improve the quality and reliability of your statistical analyses. Remember to double-check your assumptions, avoid overfitting, interpret p-values cautiously, and always report limitations. With these principles in mind, you’ll be well on your way to conducting sound and meaningful statistical research. Nu, armed with this knowledge, take the next step and apply these principles to your own work to enhance your analytical skills and decision-making capabilities. Seek out additional resources and training to continually refine your understanding of statistical methods and their proper application. Stay vigilant and committed to the pursuit of accuracy in statistical analysis to ensure the integrity of your findings and the validity of your conclusions.
Hoi, Ik ben Dieter, En ik heb Dartcounter gemaakt (Dartcounterapp.com). Mijn motivatie was geen darts -expert - helemaal tegenovergestelde! Toen ik voor het eerst begon te spelen, Ik hield van het spel, maar vond het moeilijk en afleidend om nauwkeurige scores te houden en statistieken te volgen.
Ik dacht dat ik niet de enige kon zijn die hiermee worstelde. Dus, Ik besloot om een oplossing te bouwen: een eenvoudig te gebruiken applicatie die iedereen, Ongeacht hun ervaringsniveau, zou kunnen gebruiken om moeiteloos te scoren.
Mijn doel voor Dartcounter was eenvoudig: Laat de app de nummers afhandelen - het scoren, de gemiddelden, de statistieken, Zelfs checkout suggesties - zodat spelers puur kunnen richten op hun worp en genieten van het spel. Het begon als een manier om het probleem van mijn eigen beginners op te lossen, En ik ben heel blij dat het is uitgegroeid tot een nuttig hulpmiddel voor de bredere darts -community.