Effectief stat analysis for different formats is crucial for making informed decisions, whether you’re in business, sports, or research; understanding how to tailor your analytical approach to the specific data format unlocks valuable insights. This article explores the essential techniques for conducting statistical analysis across various data types, equipping you with the knowledge to extract meaningful information from any dataset.
Still Using Pen & Paper (Of een schoolbord)?! 
Stap in de toekomst! De Dart Teller -app behandelt alle scoren, stelt kassa voor, en volgt uw statistieken automatisch. It's easier than you think!
Probeer de Smart Dart Teller -app gratis!Klaar voor een upgrade? Klik hierboven!
Understanding Different Data Formats and Their Implications for Stat Analysis
The foundation of successful statistical analysis lies in recognizing the nuances of different data formats. Data can be broadly categorized as numerical (continuous and discrete), categorical (nominal and ordinal), and text-based. Each format demands unique analytical approaches and statistical tests.
- Numerical Data: This includes continuous data (Bijv., temperature, height) and discrete data (Bijv., number of customers, number of products). It lends itself to calculations of mean, median, standard deviation, and more advanced statistical modeling.
- Categorical Data: Nominal data (Bijv., colors, types of cars) have no inherent order, while ordinal data (Bijv., customer satisfaction ratings – poor, eerlijk, good, excellent) do. Frequency distributions, chi-square tests, and other non-parametric methods are suitable for analyzing categorical data.
- Text-Based Data: Unstructured text data requires pre-processing techniques such as tokenization, stemming, and sentiment analysis before statistical methods can be applied. Techniques like text mining and natural language processing (NLP) are essential here.
Ignoring the specific characteristics of a data format can lead to inaccurate conclusions and flawed decision-making. Bijvoorbeeld, calculating the average of nominal data is meaningless.
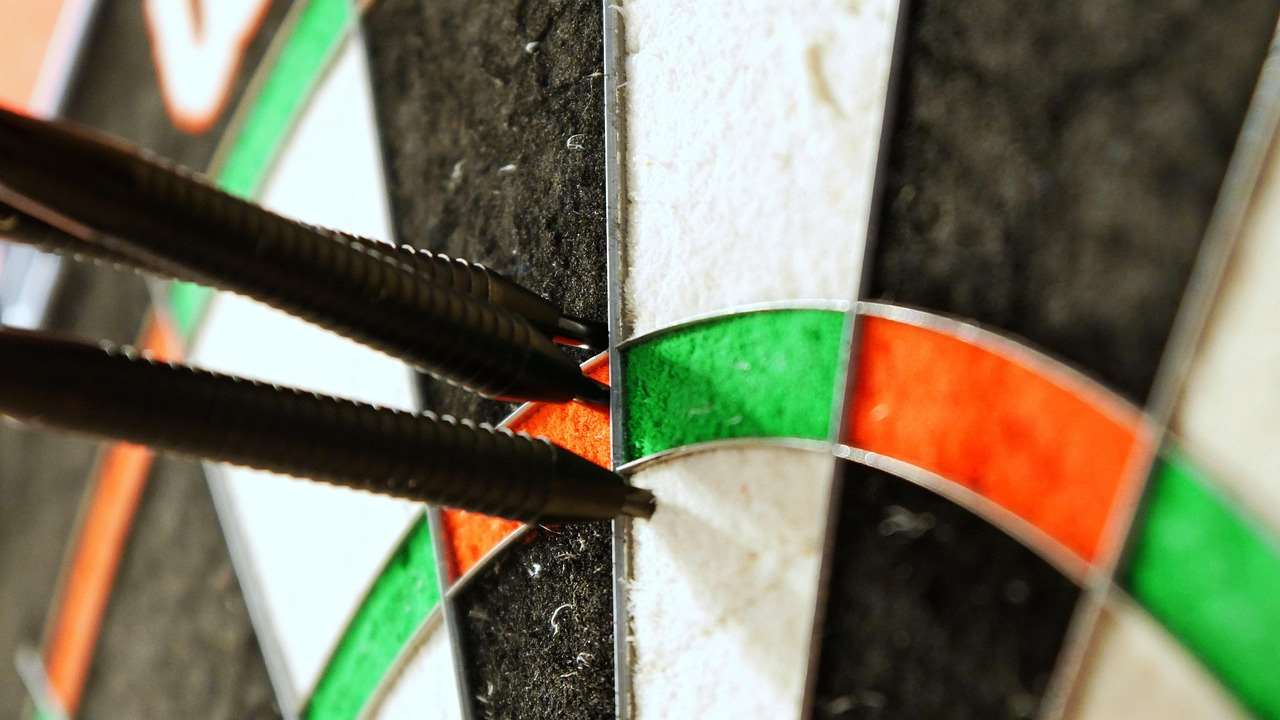
Stat Analysis For Different Formats: Choosing the Right Statistical Tests
Selecting the appropriate statistical test is paramount for drawing valid inferences from your data. The choice depends on several factors, including the data format, the research question, and the assumptions underlying the test.
Numerical Data Analysis:
For numerical data, you can employ a wide range of statistical tests, depending on your objectives:
- T-tests: Used to compare the means of two groups. There are independent samples t-tests (for comparing the means of two independent groups) and paired samples t-tests (for comparing the means of two related groups, such as before-and-after measurements).
- ANOVA (Analysis of Variance): Used to compare the means of three or more groups. ANOVA tests whether there is a statistically significant difference between the means of the groups.
- Regression Analysis: Used to model the relationship between a dependent variable and one or more independent variables. Linear regression is used when the relationship is linear, while non-linear regression is used for more complex relationships.
- Correlation Analysis: Determines the strength and direction of the linear relationship between two variables. Pearson’s correlation coefficient is used for normally distributed data, while Spearman’s rank correlation is used for non-normally distributed data.
Categorical Data Analysis:
Analyzing categorical data requires different statistical tools:
- Chi-Square Tests: Used to examine the association between two categorical variables. The chi-square test determines whether the observed frequencies differ significantly from the expected frequencies under the assumption of independence.
- Fisher’s Exact Test: An alternative to the chi-square test when sample sizes are small. It provides an exact p-value for the association between two categorical variables.
- Logistic Regression: Used to model the probability of a binary outcome (Bijv., success/failure) based on one or more predictor variables. Logistic regression is particularly useful when the dependent variable is categorical.
It is crucial to check the assumptions of each statistical test before applying it. Violating these assumptions can lead to inaccurate results. Bijvoorbeeld, many tests assume that the data is normally distributed. If this assumption is violated, non-parametric tests may be more appropriate.
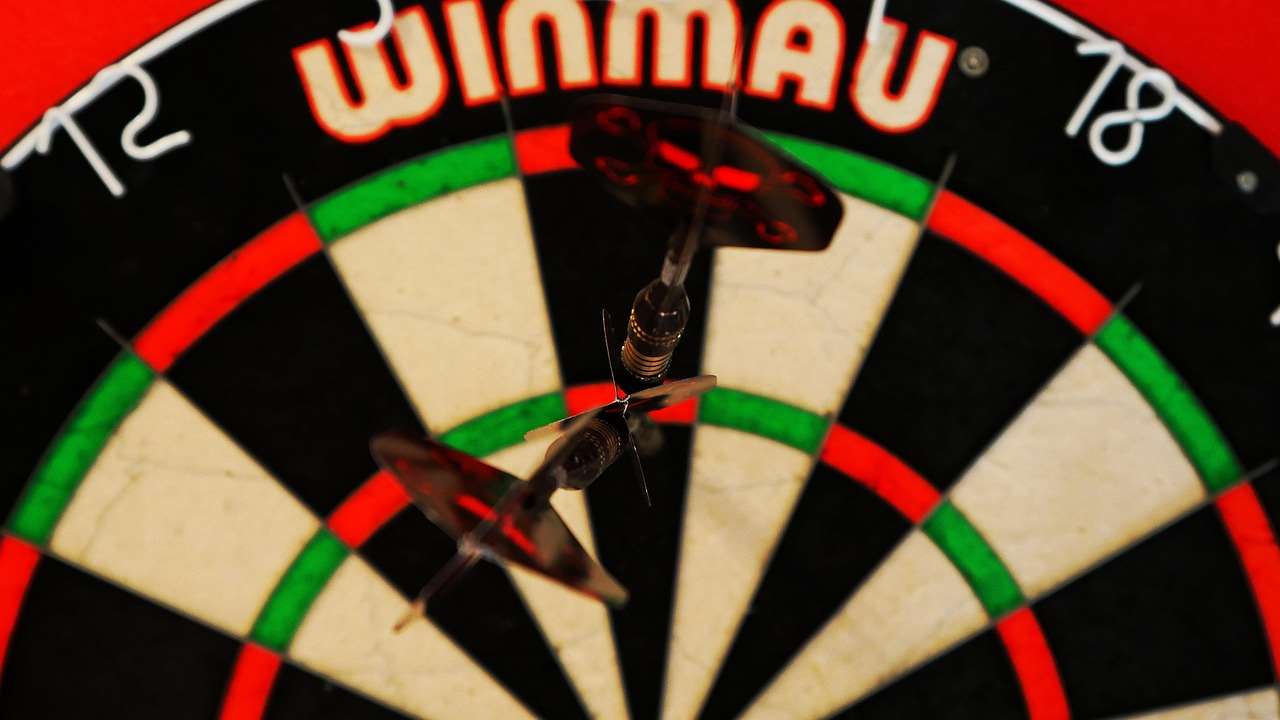
Dealing with Missing Data and Outliers in Stat Analysis For Different Formats
Missing data and outliers can significantly impact the results of statistical analysis. It’s essential to address these issues appropriately to ensure the reliability of your findings.
Handling Missing Data:
There are several approaches to handling missing data:
- Deletion: Removing observations with missing values. This can be appropriate if the amount of missing data is small and the data is missing completely at random (MCAR).
- Imputation: Replacing missing values with estimated values. Common imputation techniques include mean imputation (replacing missing values with the mean of the variable), median imputation (replacing missing values with the median), and multiple imputation (creating multiple plausible values for each missing data point).
Identifying and Handling Outliers:
Outliers are extreme values that deviate significantly from the rest of the data. They can distort statistical analyses and lead to biased results.
- Visual Inspection: Using box plots, scatter plots, and histograms to identify potential outliers.
- Statistical Methods: Using statistical tests such as the z-score or the interquartile range (IQR) method to identify outliers.
Once outliers are identified, you can choose to remove them (if they are due to errors), transform them (Bijv., using a logarithmic transformation), or use robust statistical methods that are less sensitive to outliers. Begrip Darts gok en fantasiecompetities gids might even give you an edge in spotting betting outliers.
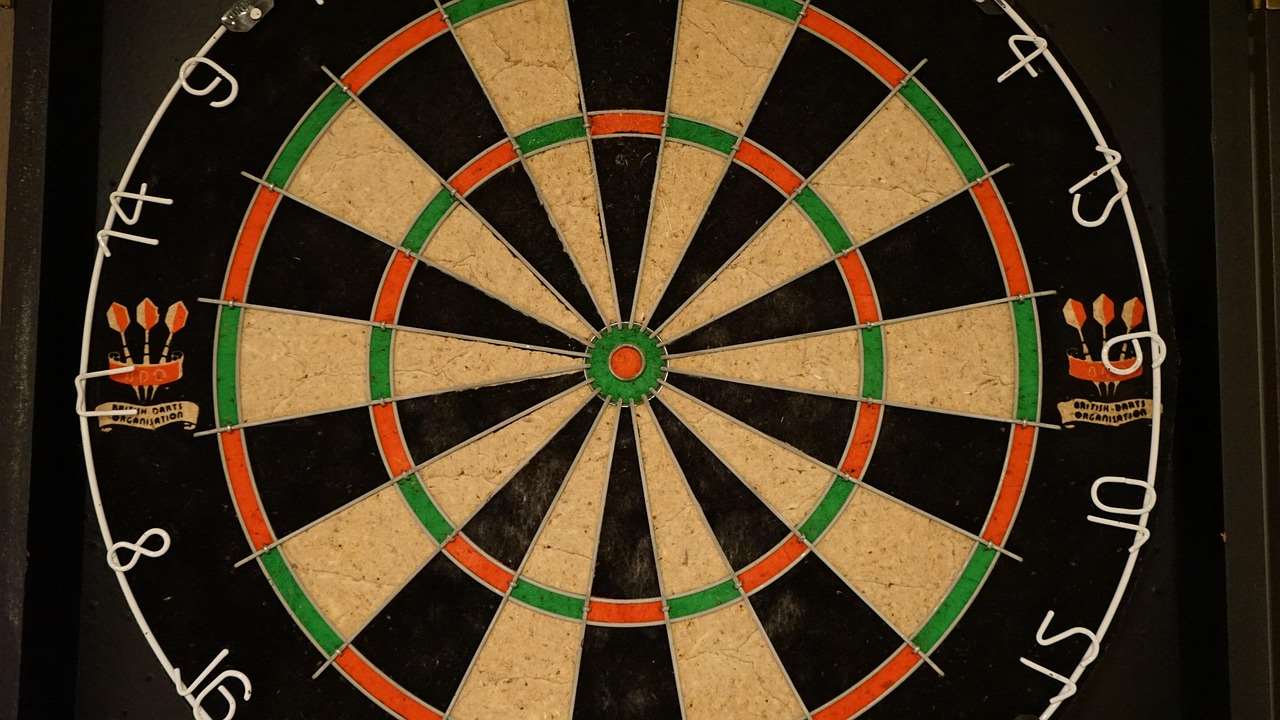
Advanced Stat Analysis Techniques: Regression and Modeling
Beyond basic descriptive statistics and hypothesis testing, advanced stat analysis techniques like regression and modeling provide deeper insights into complex relationships within your data.
Regression Analysis:
Regression analysis allows you to predict the value of a dependent variable based on one or more independent variables. Different types of regression models exist, each suited to different types of data and relationships:
- Linear Regression: Assumes a linear relationship between the dependent and independent variables.
- Multiple Regression: Extends linear regression to include multiple independent variables.
- Polynomial Regression: Models non-linear relationships by including polynomial terms of the independent variables.
- Logistic Regression: Used when the dependent variable is binary (Bijv., yes/no, success/failure).
Statistical Modeling:
Statistical modeling involves building mathematical representations of real-world phenomena. These models can be used for prediction, explanation, and decision-making.
- Time Series Analysis: Used to analyze data collected over time, such as stock prices or weather patterns.
- Cluster Analysis: Used to group similar data points together based on their characteristics.
- Factor Analysis: Used to reduce the number of variables in a dataset by identifying underlying factors that explain the correlations among the variables.
Remember to validate your models using techniques like cross-validation to ensure they generalize well to new data. Careful model selection and validation are crucial for reliable results.
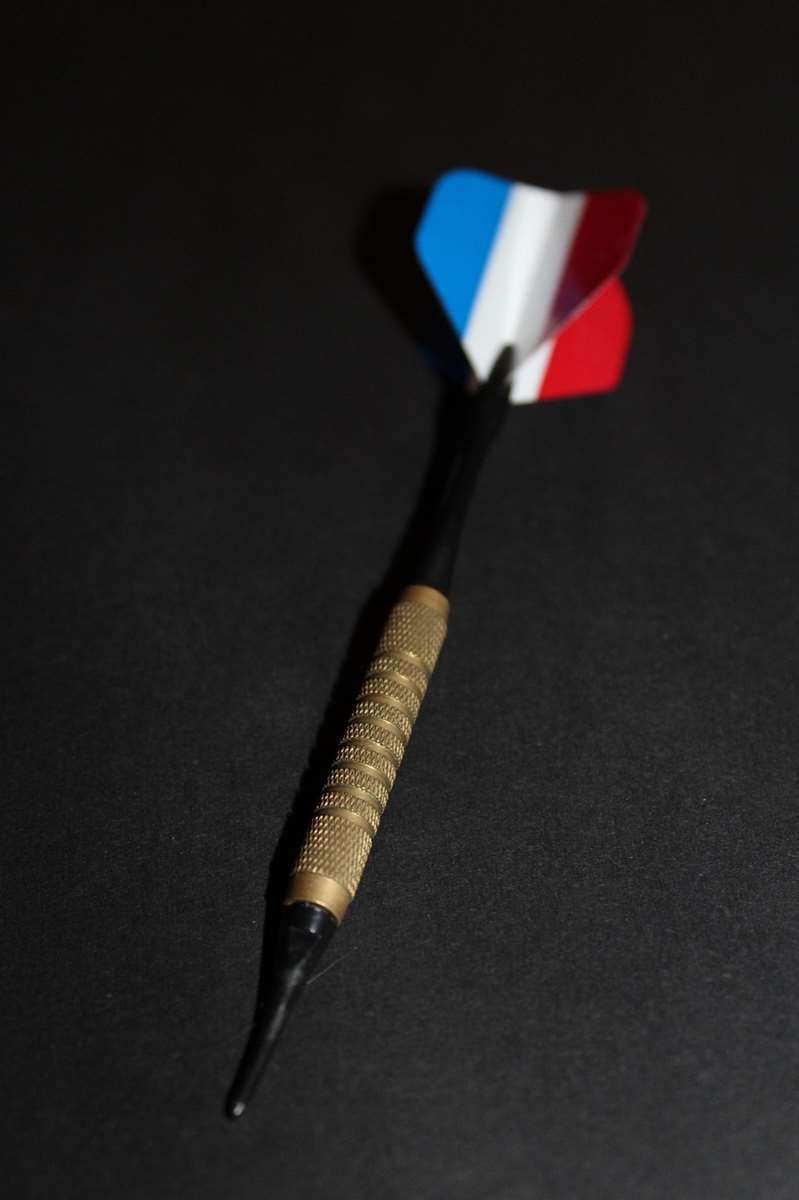
Tools and Software for Effective Stat Analysis
Numerous software packages and tools are available to assist with stat analysis for different formats, ranging from user-friendly interfaces to powerful programming environments.
- Microsoft Excel: A widely used spreadsheet program that offers basic statistical functions and charting capabilities. Suitable for simple analyses and data visualization.
- SPSS: A statistical software package known for its user-friendly interface and comprehensive set of statistical procedures.
- R: A free and open-source programming language and software environment for statistical computing and graphics. R is highly flexible and customizable, making it ideal for advanced statistical analyses.
- Python: A versatile programming language with powerful libraries for data analysis, such as NumPy, Pandas, and Scikit-learn.
- SAS: A statistical software suite widely used in business and industry.
The choice of tool depends on your specific needs, skills, and budget. Excel is a good starting point for simple analyses, while R and Python offer more advanced capabilities for complex analyses and data manipulation. Familiarize yourself with the chosen software’s documentation and online resources to maximize its potential.
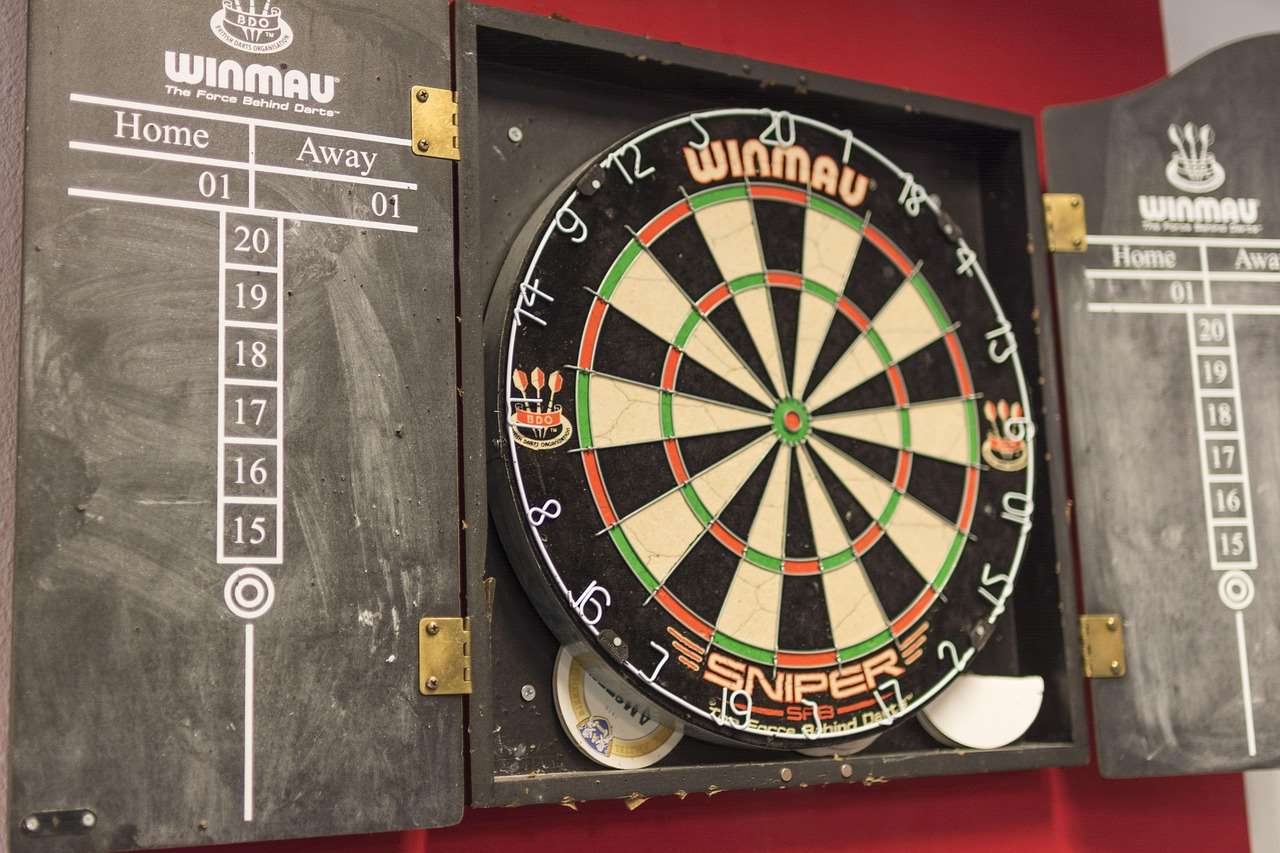
Communicating Your Stat Analysis Results Effectively
Presenting your stat analysis findings clearly and concisely is just as important as performing the analysis itself. Effective communication ensures that your insights are understood and acted upon.
Data Visualization:
Use charts and graphs to illustrate your key findings. Choose the appropriate type of visualization based on the data and the message you want to convey. Common visualization techniques include:
- Bar charts: For comparing categorical data.
- Line charts: For showing trends over time.
- Scatter plots: For showing the relationship between two numerical variables.
- Histograms: For showing the distribution of a numerical variable.
- Box plots: For displaying the distribution of data and identifying outliers.
Clear and Concise Writing:
Use clear and concise language to describe your findings. Avoid jargon and technical terms that your audience may not understand. Focus on the key takeaways and their implications.
Structured Reporting:
Organize your report logically, with a clear introduction, methodology, results, and conclusion. Include tables and figures to support your findings. Clearly label all axes, tables, and figures.
Consider your audience when preparing your report. Tailor the content and language to their level of understanding. Visuals can often simplify complex information, enabling Bookmaker Sponsorship Professional Darts to be better understood.
Conclusie
Mastering stat analysis for different formats empowers you to extract actionable insights from diverse datasets. By understanding the nuances of numerical, categorical, and text-based data, selecting appropriate statistical tests, handling missing data and outliers, and effectively communicating your findings, you can make data-driven decisions that drive success. Remember to choose the right tools, software, and visualization techniques to present your analyses effectively. Continuously refine your analytical skills and stay updated with the latest advancements in the field of statistics. Ready to put these principles into practice? Start by identifying a dataset relevant to your field and applying the techniques discussed in this article. Embrace the power of data to unlock valuable knowledge and make informed choices!
Hoi, Ik ben Dieter, En ik heb Dartcounter gemaakt (Dartcounterapp.com). Mijn motivatie was geen darts -expert - helemaal tegenovergestelde! Toen ik voor het eerst begon te spelen, Ik hield van het spel, maar vond het moeilijk en afleidend om nauwkeurige scores te houden en statistieken te volgen.
Ik dacht dat ik niet de enige kon zijn die hiermee worstelde. Dus, Ik besloot om een oplossing te bouwen: een eenvoudig te gebruiken applicatie die iedereen, Ongeacht hun ervaringsniveau, zou kunnen gebruiken om moeiteloos te scoren.
Mijn doel voor Dartcounter was eenvoudig: Laat de app de nummers afhandelen - het scoren, de gemiddelden, de statistieken, Zelfs checkout suggesties - zodat spelers puur kunnen richten op hun worp en genieten van het spel. Het begon als een manier om het probleem van mijn eigen beginners op te lossen, En ik ben heel blij dat het is uitgegroeid tot een nuttig hulpmiddel voor de bredere darts -community.